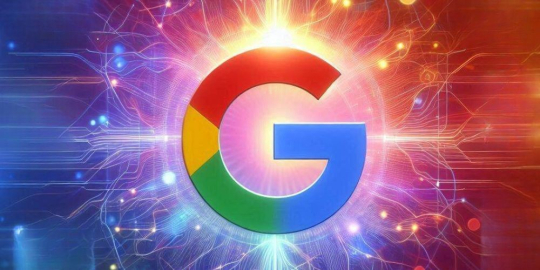
Back in February, Google faced significant backlash over its AI-powered chatbot Gemini’s flawed ability to generate accurate images of people. Users were quick to point out historical inaccuracies and racial stereotypes, such as the anachronistic depiction of a "Roman legion" and stereotypical portrayals of "Zulu warriors." These misrepresentations prompted a public apology from Google CEO Sundar Pichai, and DeepMind co-founder Demis Hassabis assured users that a fix would arrive swiftly. However, despite these promises, the issue remains unresolved well into May.
At the recent annual I/O developer conference, Google showcased various Gemini features, from custom chatbots and vacation planners to integrations with Google Calendar and YouTube Music. Yet, conspicuously missing was any update on the image generation of people. A Google spokesperson confirmed that this feature continues to be disabled in Gemini apps on both web and mobile platforms. The problem, it seems, is far more complicated than initially suggested by Hassabis.
The crux of the issue lies in the datasets used to train image generators like Gemini's. These datasets predominantly feature images of white individuals, with comparatively fewer images of people from other races and ethnicities. Furthermore, the images of non-white individuals often perpetuate harmful stereotypes. In an attempt to rectify these biases, Google employed a clumsy hardcoding mechanism, which has proven ineffective and problematic. Now, the company is grappling with finding a balanced solution that addresses these concerns without repeating historical inaccuracies.
Will Google eventually find a fix for Gemini's biased image generator? It's hard to say. The drawn-out nature of this issue highlights just how challenging it is to correct biases inherent in AI systems. Bias is deeply ingrained and multifaceted, requiring a nuanced and carefully calibrated approach to resolve. This case serves as a vivid reminder of the difficulties involved in developing ethical and accurate artificial intelligence technologies.
In conclusion, Google's struggle with Gemini's image generation highlights a significant challenge in AI development: addressing and rectifying biases while striving for accuracy. While the company has made strides in other areas, the failure to resolve this particular issue underscores the intricate difficulties involved. As Google continues to work on a solution, this incident remains a potent example of the need for continued vigilance and improvement in AI ethics and development practices.